포스팅 개요
본 포스팅은 추천 시스템(RecSys, recommender system) 논문들 중 Multi-Interactive Attention Network for Fine-Grained Feature Learning in CTR Prediction (MIAN) 이라는 논문을 리뷰하는 포스팅입니다. 본 논문의 추천 시스템은 attention model을 활용을 하면서 다양한 interaction attention layer를 도입합니다. 이를 활용해 user sequential 한 상황에서 different feature에 대한 multiple representation을 활용할 수 있게 제안하며 fine grained user spedific 정보 및 context 정보도 동시에 활용하도록 제안하는 추천 시스템 논문입니다.
또한, 본 포스팅은 제가 작성하고 있는 추천 시스템 시리즈 글 입니다. 시리즈로 작성하는 추천 시스템 논문 리뷰는 아래와 같은 순서로 진행할 예정입니다. 오늘은 그 여덟 번째 MIAN 포스팅입니다.
- DeepFM (https://lsjsj92.tistory.com/636)
- MAML (https://lsjsj92.tistory.com/637)
- VECF (https://lsjsj92.tistory.com/638)
- FDSA (https://lsjsj92.tistory.com/639)
- PMN (https://lsjsj92.tistory.com/640)
- GAU (https://lsjsj92.tistory.com/641)
- A3NCF(https://lsjsj92.tistory.com/642)
- MIAN
- CCANN (https://lsjsj92.tistory.com/644)
본 포스팅에서 참고한 자료는 다음과 같습니다.
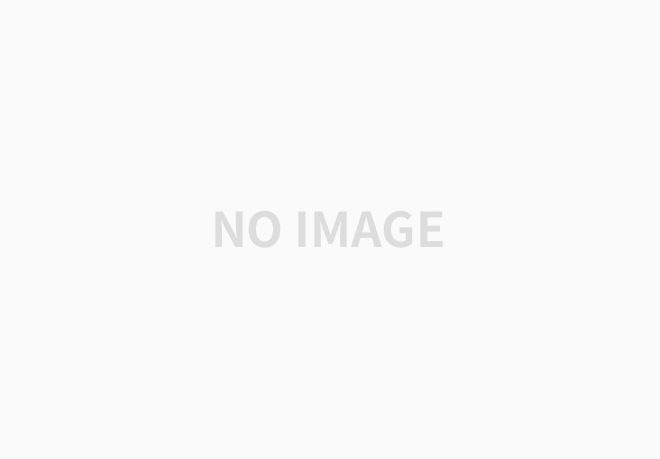
포스팅 본문
본 포스팅의 Multi Interactive Attention Network for Fine grained Feature Learning in CTR Prediction(MIAN) 추천 시스템 논문 리뷰는 다음과 같은 순서로 진행합니다.
1. MIAN 핵심 요약
2. MIAN 논문 리뷰
MIAN 핵심 요약
기존 CTR prediction는 user sequential 행동을 잘 활용하지만, 한계가 존재
1. 기존 방법은 대부분 사용자 행동을 활용
• 사용자는 과거 행동과 무관한 새 제품을 종종 클릭하는데 이런데 적합하지 않음
2. real scenario에서는 수많은 사용자들이 오래전에 활동함
• 최근에는 상대적으로 비활성화 됨
3. 사용자 이력에서 multiple representation in different feature subspace는 무시
본 논문은 MIAN(Multi-Interactive Attention Network)을 제안
• 모든 종류의 fine-grained feature 사이의 latent relationship을 포괄적으로 추출
• Multi Interactive Layer를 도입
• 3개의 local interaction module를 통합
• Sequential 행동에서 multiple representations of user preference를 캡처
• Fine-grained user specific 정보를 활용할 뿐만 아니라 컨텍스트 정보도 동시에 활용
• Global Interaction Module(GIM)을 도입
• High order interaction을 학습
• multiple feature의 서로 다른 impact의 균형을 잡아줌
MIAN 논문 리뷰
Multi-Interactive Attention Network for Fine-Grained Feature Learning in CTR Prediction (MIAN) 논문에 대한 리뷰와 정리를 시작합니다. 본 포스팅은 논문에 나와 있는 순서대로 정리합니다.
Introduction
추천 시스템에서 CTR의 약간의 상승은 revenue에 huge impact를 가져옴
Factorization Machine, Logistic Regression 등의 노력과 연구가 있었습니다. 이 연구들의 특징은 Shallow feature combinations입니다. 최근에는 Deep learning을 추천 시스템에 많이 활용하고 적용하였습니다. 그 예시로 Wide & Deep, Deep & Cross(DCN), DeepFM 등이 있죠. 딥러닝을 활용한 추천시스템은 다양한 방법이 있지만 그 중 Sequential 방법을 활용한 Recurrent Neural Network based 연구도 진행이 되었습니다. 이는 사용자의 historical behavior를 통해 user interest representation을 추출하며 대표적으로 Deep Interest Net(DIN), DIEN 등이 있습니다. 이렇게 딥러닝을 활용한 추천 시스템 방법은 high order interest이고 이를 활용하면 model representation 능력을 향상시키고 CTR에도 도움을 준다고 합니다.
하지만, 한계가 존재
본 논문에서는 Fine-grained user specific 정보, context 정보에는 little attention하다고 합니다. 그것에 대한 설명은 다음과 같습니다.
• 사용자 historical 행동과 irrelevant한 새로운 요구를 자주 가지고 있기에 CTR 예측을 mislead할 수 있음
• CTR 시나리오에서 많은 사용자의 클릭 행동은 대부분 오래전에 발생하고 최근 기간에는 activites가 부족함
• 사용자 과거 행동에만 의존하면 사용자의 현재 요구와 맞지 않은 outdated recommendation을 lead할 수 있음
• Sequential behavior encoding의 경우 diversity of feature interaction in different subspace가 완전히 고려되지 않음
• Click-through context에 따른 historical behavior의 표현은 달라야 함
User specific and context information
추천 시스템 쪽에는 user spedific한 정보와 context 정보가 많이 있다고 본 논문에서는 이야기 하고 있습니다. 이러한 Fine-grained information은 사용자의 현재 상태를 유추할 수 있는 단서를 제공하고 개인화 성능을 향상시킬 수 있습니다.
• 사용자의 현재 직업 ( 프로그래머 )등이 유추 가능하며 이는 과거 이력에서는 표현하기 어려울 수 있는 것임
• 이렇게 fine-grained feature interaction을 사용하면 현재 preference of user를 모델링 하는데 도움
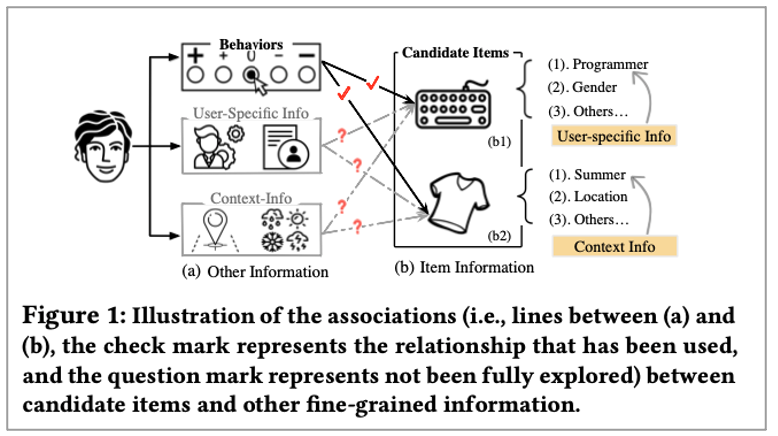
따라서, 본 논문에서는 MIAN 이라는 모델을 제안!
이에 본 논문에서는 Multi Interactive Attention Network(MIAN)이라는 추천 시스템 모델을 제안합니다. 본 논문에서 제안한 모델은 Fine-grained feature와 candidate item 사이의 latent representation을 얻을 수 있고 multiple information을 집계하는 특징을 가지고 있습니다. 또한, Multi Interactive Layer(MIL)이라는 것을 도입하는데요. 그 특징은 다음과 같습니다.
• 3개의 local interaction module과 global interaction module을 포함한 layer
• 첫 번째 local module : Item Behaviors Interaction Module (IBIM)
• Different subspace에서 sequential 동작의 사용자 선호도를 Adaptively explore 하기 위해 Pre-LN Transformer를 사용
• 두 번째 local module : Item User Interaction Module (IUIM)
• Candidate items과 fine-grained user specific information 사이의 knowledge를 capture하는 것이 목표
•세 번째 local module : Item Context Interaction Module (ICIM)
• Candidate item과 context aware information 사이의 관계를 파악하기 위해 고안
• Global Interaction Module (GIM)
• Embedding 이후 low order feature와 3개의 local module에서 나온 high order feature 사이의 weigh the influence를 study하기 위해 고안
Main contributions
본 논문에서 주장하는 contribution은 다음과 같습니다.
1. User historical behavior와 multiple fine grained feature interaction의 문제를 동시에 연구
• 이전 CTR 방법에 의해 explicitly 그리고 jointly하게 모델링 되지 않은 사용자의 이력에서
2. MIAN 모델을 제안
• Fine grained feature interactive learning을 위한 Multi-interactive layer를 포함
• Different feature subspace에서 multiple representations of user behavior를 추출하기 위한 Transformer 기반의 module 포함
• 3. Extensive experiments
• 3개의 large dataset
• State of the art를 달성했고 model explainability도 좋음
• Large commercial system에서 significant improvement를 달성
Related Work
본 논문과 연관된 다양한 추천 시스템 논문들이 있습니다. 본 포스팅에서는 간단하게만 정리합니다. 자세한 것은 논문을 확인해주시면 더 좋을 것 같습니다! 연관된 연구 중 Shallow model 기반이 있습니다. 여기에는 Logistic Regression(LR), Gradient Boosting Decision Trees(GBDT)이 해당됩니다. Factorization Machine(FM) 계열도 있는데요. FM 계열에는 FM, Attentional Factorization Machine(AFM), Neural Factorization Machine(NFM) 등이 해당됩니다. Deep Learning model기반에는 Wide & Deep, Deep & Cross network(DCN), DeepFM, xDeepFM 등이 해당됩니다.
본 논문에서는 위 연구들의 한계점이 존재하며, 이를 해결하기 위해 MIAN이라는 것을 제안한다고 합니다. 그 한계는 다음과 같습니다.
• sequential behavior information을 무시함
• 과거 사용자의 행동은 사용자의 선호도를 explicit하게 나타내기 때문
• DIEN, DIN 등은 이런 sequential behavior의 중요성을 알고 활용함
• 기존 sequential based model
• Other information을 one vector에 투영
• 동등한 attention을 candidate item과 fine-grained information 사이에 반영하지 않음
따라서 위 문제를 해결하기 위해 본 논문에서는 Sequential behavior와 other fine-grained information을 동시에 탐색하도록 모델을 제안합니다.
The MIAN Model - Problem Definition
이제 본격적으로 본 논문에서 제안하는 추천 시스템 모델에 대한 설명을 진행합니다. 본 논문에서는 CTR prediction으로 문제를 정의하였습니다. 그 중에 Sequential 기반에 집중했는데요. Sequential CTR model은 4개의 groups of features로 input이 들어온다고 합니다. 그 groups of feature는 다음과 같은 형태입니다.
• CTR = f(candidate itme, behaviors, user profile, context)
또한, Group에서 fine-grained feature를 활용한다고 합니다.
• user specific fields : gender, age, occupation, season 등
• Context fields : log time, location 등
더불어서 본 논문에서는 Attention mechanism을 활용해 fine grained feature와 candidate item을 모델링한다고 합니다. 위에서 설명드린 각 표기법은 다음과 같습니다.
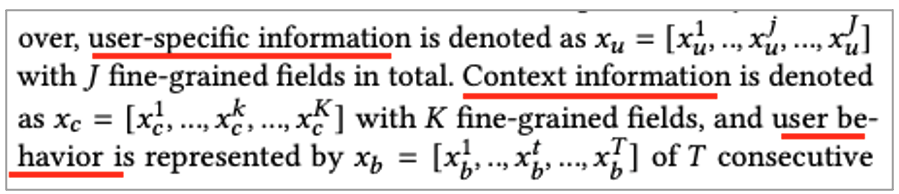
• User behavior는 연속적인 sequential behavior임
The MIAN Model - Components of MIAN
본 논문에서 제안한 모델인 MIAN은 다양한 컴포넌트가 있습니다. 이번 파트에서는 MIAN의 component들을 설명합니다. 먼저 MIAN은 크게 3개의 layer가 포함된다고 하며 이는 다음과 같습니다.
• Embedding Layer, Multi Interactive Layer, Prediction Layer
3개의 layer와 더불어서 4개의 raw data group이 있으며 이는 다음과 같습니다.
• Candidate item, historical behavior, user specific, context information
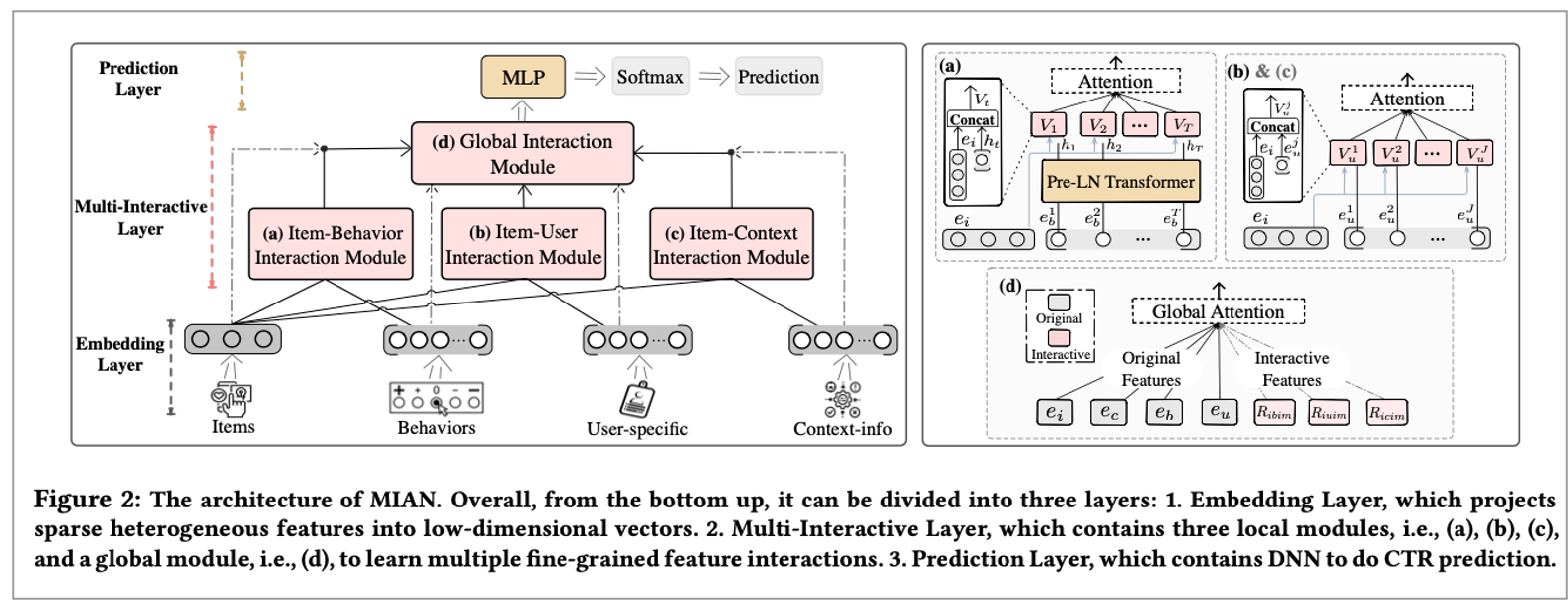
위에서 3개의 layer(embedding, multi interactive, prediction)가 있다고 언급했는데요. 각 layer에 대해서 조금 더 자세히 살펴보겠습니다.
1. Embedding Layer
• High dimensional을 low dimensional space에 projected(투영)함

2. Multi Interactive Layer ( MIL )
• Candidate item과 other information관계를 효과적으로 모델링하는 layer
• 4개의 Module이 존재함
• Item Behaviors Interaction Module ( IBIM )
• Item User Interaction Module ( IUIM )
• Item Context Interaction Module ( ICIM )
• Global Interaction Module ( GIM )
이제 이 Multi Interactive Layer안에 있는 모듈(module)을 자세히 알아보도록 하겠습니다.
2-1. Multi Interactive Layer – Item Behaviors Interaction Module (IBIM)
Multi Interactive Layer의 Item Behaviors Interaction Module(IBIM) 모듈에서는 Candidate item과 user sequential behavior information을 attention mechanism을 이용해 동시에 모델링합니다. 여기서의 특징은 Model의 complexity 및 amount of parameter를 RNN based 보다 상대적으로 작게 합니다. 또한, 선행 연구가 된 Transformer보다 더 빠르고 안정적이게 수렴하는 Pre-LN Transformer를 사용합니다.
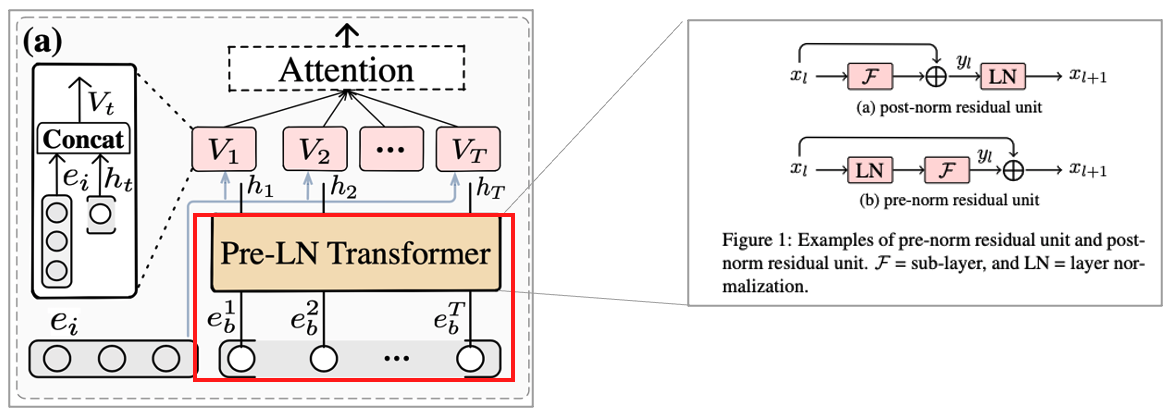
해당 모듈에서 모델의 형태는 다음과 같은 형태를 가지고 있습니다.
• Layer norm, multi head self attention, position wise feed forward network를 stacking한 것
• Layer norm을 multi head self attention 및 position wise feed forward network 전에 적용함
• Multi head self attention을 이용해 multiple subspace representation을 학습할 수 있음
• 또한, Multi head self attention은 일련의 linear transformation이기 때문에 FFN을 통해 non-linear를 적용함
• 여기까지 하면, input e_b에 따른 h_b[h_1 … h_t]의 Pre-LN Transformer unit이 나오게 됨
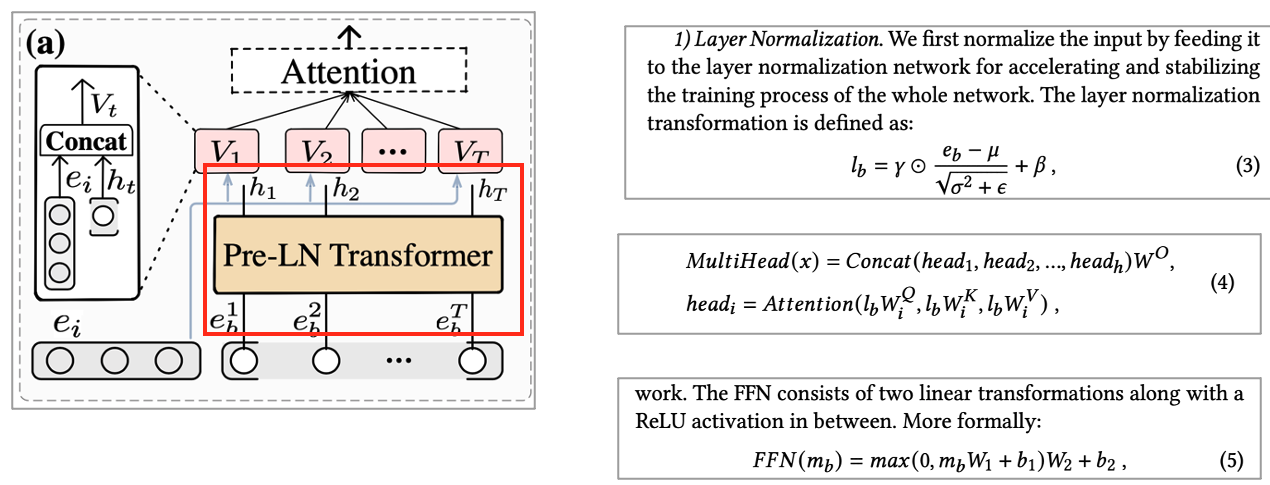
Pre-LN unit에서는 User behavior의 hidden state에 대해 candidate item과 간단하게 concat합니다. 이를 통해 각 시간 step에서 interactive feature representation을 얻을 수 있습니다. 또한, 사용자의 다양한 흥미는 시간에 따라 달라지기에 history behavior는 관심 representation에 다른 영향을 미칠 수 있습니다. 즉, 해당 기여도를 정량화 할 필요가 있는데, 어텐션 매커니즘(attention mechansim)으로 가중치를 강조할 수 있다고 논문에서는 이야기합니다. 이 방법은 다음과 같습니다.
• Concat한 것에 attention weight를 구함으로써 추가로 user interest representation을 얻을 수 있음
• 이를 V_t에 weighted sum 함으로써 output 제공
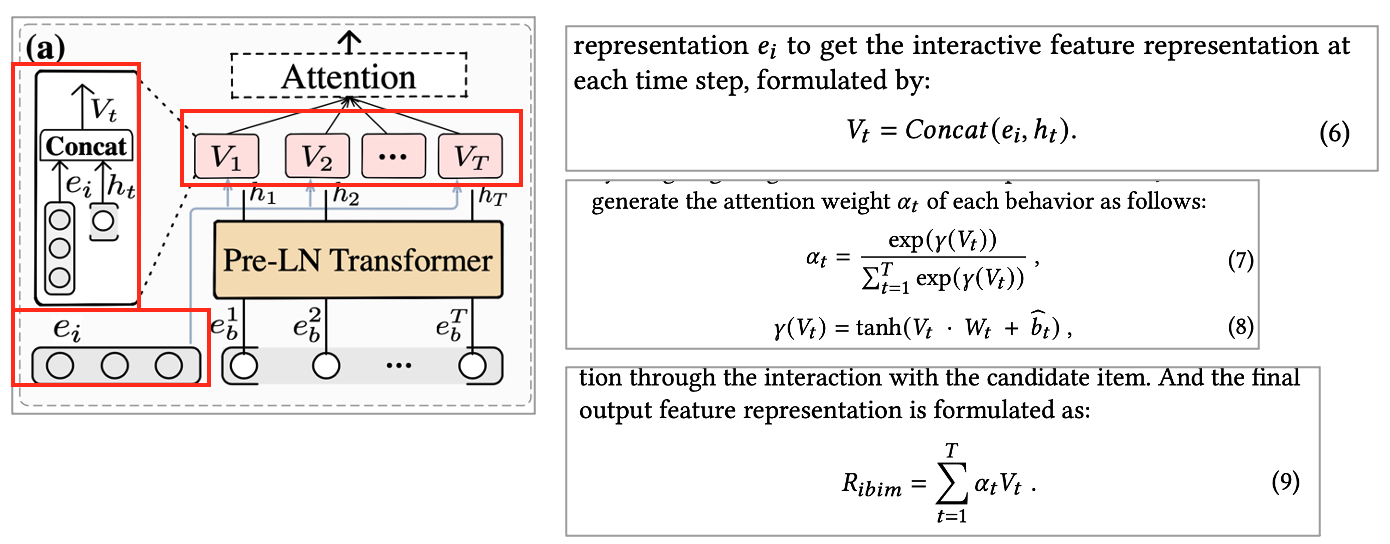
2-2. Multi Interactive Layer – Item-User Interaction Module (IUIM)
Multi Interactive Layer의 Item User Interaction Module(IUIM)에서는 Candidate item은 user-specific information과 연관될 수 있다고 가정합니다. 이때 User sequential behavior에서 user preference가 누락될 수 있으므로 user specific information으로 보완합니다. 왜냐하면 기존에는 단순히 historical behavior에만 초점을 맞추어서 제한이 있었기 때문입니다.
즉, Candidate item과 find-grained user specific information 사이의 interactions를 마이닝하기 위해 IUIM 도입합니다. IUIM 모듈에서는 다음과 같이 수행합니다.
• Item representation e_i와 fined grained user specific representation을 concat
• 그리고 attention weight를 구함
• candidate item과 가장 잘 일치하는 fine grained user specific information을 학습하고 추출할 수 있음
• Output은 weighted sum한 것

2-3. Multi Interactive Layer – Item-Context Interaction Module(ICIM)
Fine-grained context information과 candidate 사이의 interaction은 중요한 요소이므로 Multi Interactive Layer의 Item Context Interaction Module(ICIM)에서는 이 부분에 집중합니다. 이전 연구들에서는 해당 정보에 크게 집중하지 않았다고 논문에서 이야기 하며 시즌, 날씨 등의 정보 등이 여기에 해당 된다고 합니다. ICIM은 IUIM과 비슷한 형태로 scaled attention representation 부여합니다.
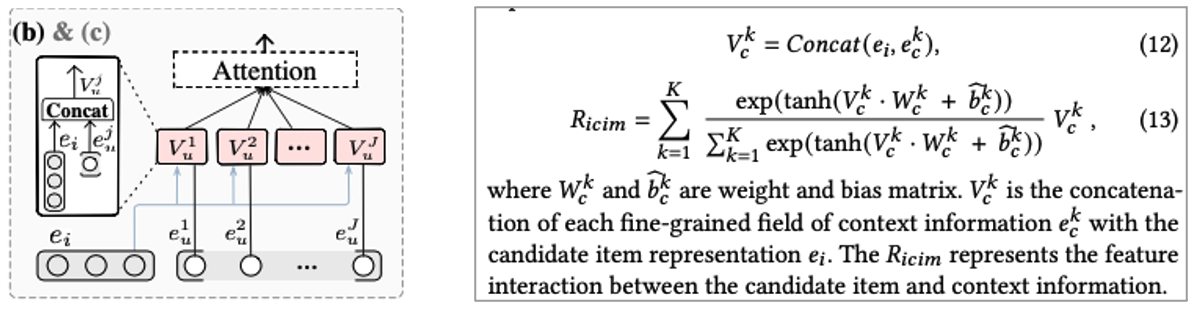
2-4. Multi Interactive Layer – Global Interaction Module (GIM)
Original feature representation과 앞서 생성한 interactive feature representation을 얻을 수 있습니다. 이전 Deep&Cross 연구에서 Low order와 high order feature의 interaction의 중요성이 주장되었었는데요. 여기서 아이디어를 얻어 Multi Interactive Layer의 Global Interaction Module(GIM)에서는 original feature(low order) interaction feature(high order)의 관계를 활용합니다. Different interaction module과 original embedding에서 중요성을 추출하기 위해 attention mechanism 활용하며 All feature를 concat하고 attention 수행합니다.
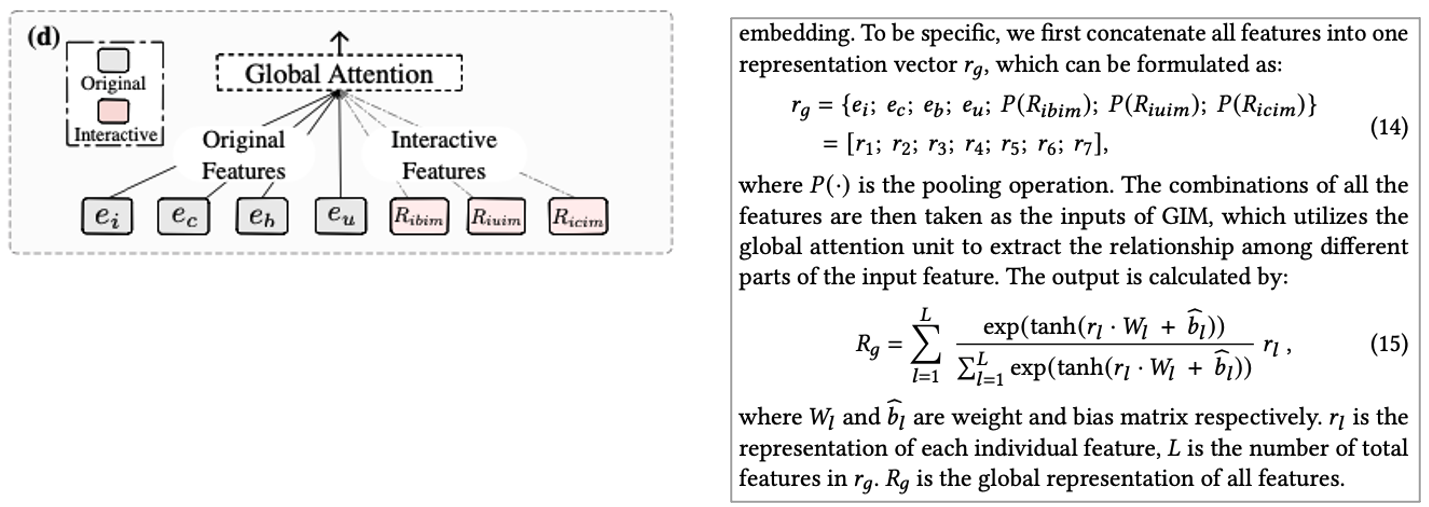
3. Prediction Layer
layer에 대한 설명중 마지막으로 Prediction layer에 대해 정리하면
• 앞서 생성한 global feature representation R_g가 DNN prediction module로 들어가 CTR prediction 수행
• Non-linear layer를 쌓고 high-order interaction을 학습
• 마지막엔 softmax
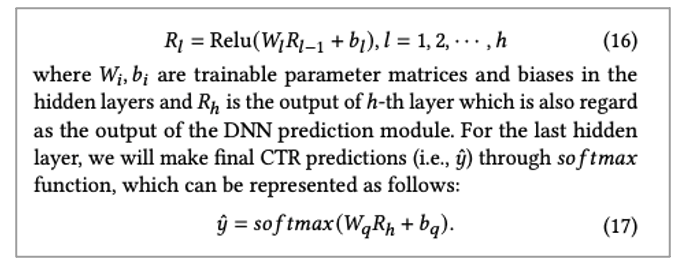
이 됩니다.
The MIAN Model - Training Strategy
본 논문에서 제안하는 training 전략은 cross entropy를 활용하는 것입니다.
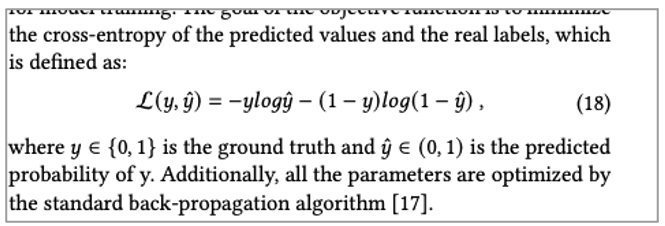
Experiments - Datasets Description
본 논문에서 사용한 데이터는 Amazon Dataset과 Commercial Dataset입니다. 그 중 Commercial Dataset은 Alipay recommendation system에서 나온 30일치 click log 데이터를 활용했다고 하며 candidate item, user specific, context, historical behavior 등이 포함되어 있다고 합니다. 이 commercial dataset의 특징은 다음과 같은 것들이 있습니다.
• Real ctr scene의 현상을 볼 수 있음
• 1. 과거 동작과 irrelevant한 candidate item
• 2. 사용자 동작의 50%는 30일 전에 발생
• 3. commercial에서 fine-grained user specific and context information이 많이 있음
• 대조적으로 user sequential behavior는 약간 불충분
• 이러한 것들이 본 논문에서 제기 했던 문제들임
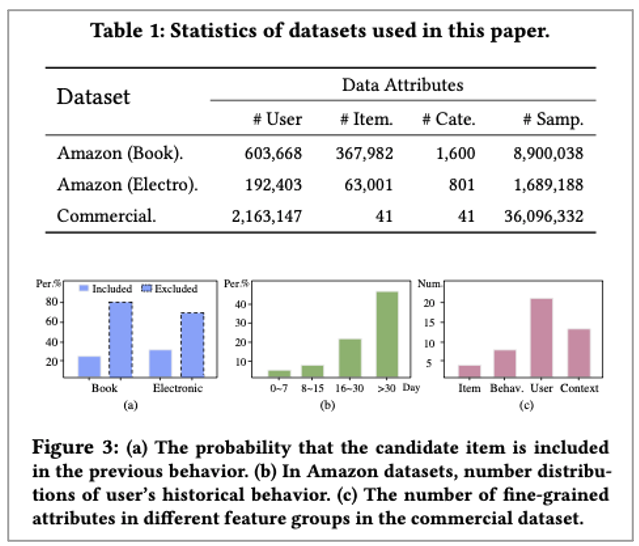
Experiments - Baseline Methods
MIAN 추천 시스템 논문에서 사용한 baseline 방법들은 기존에 wide하게 사용되는 sota 모델들을 활용합니다. 사용한 모델들은 다음과 같고 DeepCTR pytorch를 활용했다고 합니다.
• Linear Base : Factorization Machine
• Wide & Deep
• Deep & Cross
• DeepFM
• xDeepFM
• DIN
• DIEN
Experiments - Experiments Setups
실험 환경은 논문에 자세히 나와있습니다. 본 포스팅에서는 간단하게 해당되는 논문 내용만 올려두겠습니다.
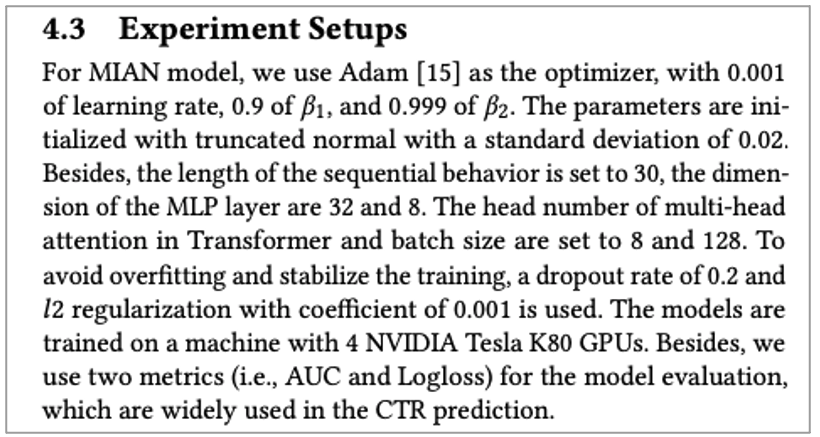
Experiments - offline Experimental Result
이제 각 실험에 대한 결과를 보여줍니다. 실험 결과 중 offline metric에 대한 결과를 먼저 정리합니다. 그 결과는 아래와 같습니다.
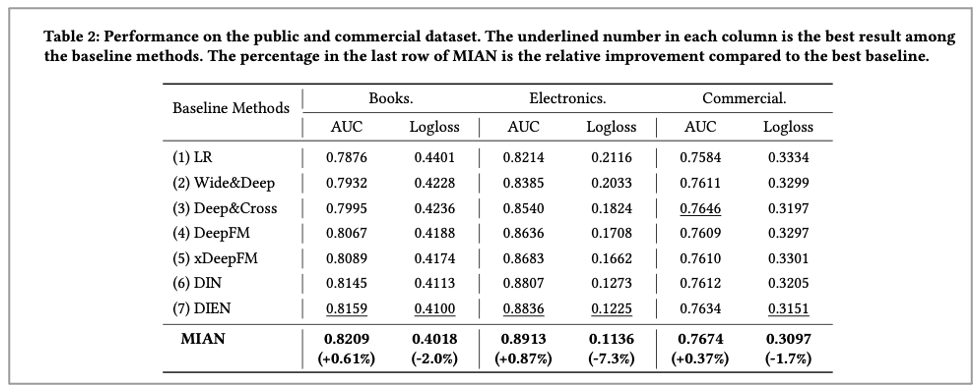
1. Amazon dataset
• DIN, DIEN이 XDeepFM과 다른 baseline 보다 좋은 성능
• 이들은 non sequential model로 user historical behavior와 candidate item과의 interaction을 무시하기 때문
• 즉, Sequential behavior가 꽤나 중요하다는 것을 의미
2. MIAN은 SOTA 달성
• DIEN 등보다 좋은 성능
• Fine grained multiple interaction of historical behavior, user specific and contextual interaction을 학습하기 때문
• DIEN은 sequential behavior만 집중
• Transformer를 활용해 different subspace로 internal dependency를 capture
3. Commercial dataset
• Sequential behavior는 부족하지만 fine grained information 존재
• DCN이 DIEN보다 약간 우수한데, multiple feature interaction을 고려하기 때문
• 여전히 MIAN이 우수한 성능
• User sequential behavior와 fine grained feature interaction을 동시에 학습하기 때문
4. MIAN의 효과에 대한 관점
• 1. Transformer를 활용한 sequential behavior interaction의 효과
• 2. attention mechanism으로 fine grained user specific and context feature interaction의 충분한 활용
• 3. global weighting과 original representation 그리고 high level interactive 표현을 통합한 것
이렇게 정리될 수 있습니다.
Experiments - Model Efficiency
모델의 Efficiency을 테스트 해보니 DIN, DIEN 등은 LR < Wide&Deep < DeepFM < DIN < DIEN으로써 paper에서 나온 것처럼 같은 트랜드를 확인할 수 있었으며 본 논문에서 제안한 추천 시스템 모델 MIAN의 Efficiency는 Computation time of MIAN 기준과 Per epoch 기준, 4 NVIDIA Tesla K80 GPU 기준으로 다른 popular sequential model과 비슷한 결과를 볼 수 있었다고 합니다. 그 특징은 다음과 같습니다.
• DIN : 3,143 seconds
• MIAN : 3,700s
• DIEN : 4,073s
여러 unit들의 결합으로 DIN보단 느리지만, DIEN보단 빠르며 이는 GPU의 병렬처리로 가능한 것이라고 합니다.
Experiments - Online A/B Testing Result
본 논문에서는 offline test외에도 online test도 진행했습니다. 이를 위해 A/B test를 진행하였으며 Alipay에서 10일동안 A/B testing을 진행하여 MIAN의 effectiveness를 설명합니다. 이때 A/B test 목표는 현재 best 모델인 DCN과 비교하여 CTR을 늘리는 것을 목표로 정하였습니다.
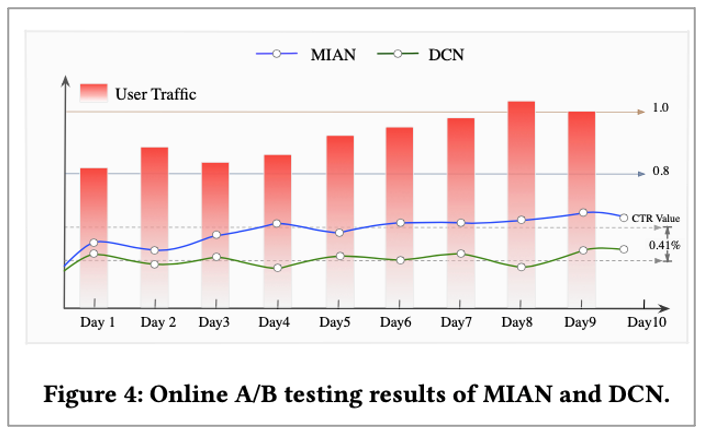
A/B Test를 해본 결과 0.41% CTR이 증가하고 COST는 0.27% 감소시켰다고 합니다. 본 논문에서는 Large scale commercial platform에서는 0.1% 상승이 큰 이익을 가져오기 때문에 이는 비즈니스 수익 증가에 통계적으로 유의하다고 주장합니다.
Experiments - Ablation Study and Visualization Study
위 실험들 외에도 다양한 ablation study와 visualization study도 진행하였습니다. 먼저 Ablation study에서는 multi interactive layer에 대해서 다양하게 study 해봤으며 그것에 대한 설명은 다음과 같습니다.
1. Abldation study - Effectiveness of Multi-Interactive Layer
본 실험에서는 Multiple ablation study로 each component의 effectiveness를 실험하였습니다. 즉 IUIM, ICIM, IBIM 등을 빼고 진행하는 것 등의 실험을 진행하였습니다.
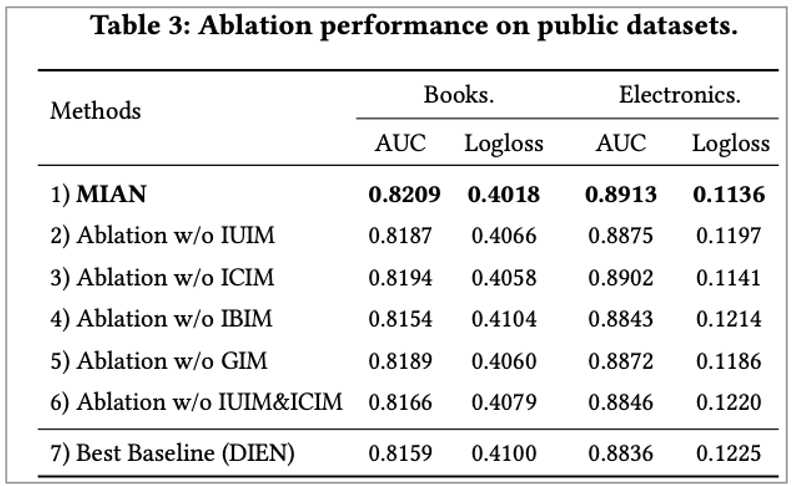
실험 결과는 다음과 같이 정리될 수 있습니다.
• IBIM제거가 성능 타격에 가장 큰 영향
• MIAN이 user historical preference 추출에 sequential behavior가 중요하다는 것을 알 수 있음
• IUIM, ICIM도 제거 시 성능 저하를 보여줌
• User-specific, context specific의 영향
• 이런 extreme setting에도 불구하고 DIEN을 뛰어넘는 성능
• 이전 sequential method보다 효과적, 충분하게 활용한다는 것을 시사
• GIM 제거도 약간의 성능 저하를 보여줌
2. Abldation study - Effectiveness of Pre-LN Transformer
본 실험에서는 Pre-LN Transformer 방식의 효과를 보여주는 실험입니다.
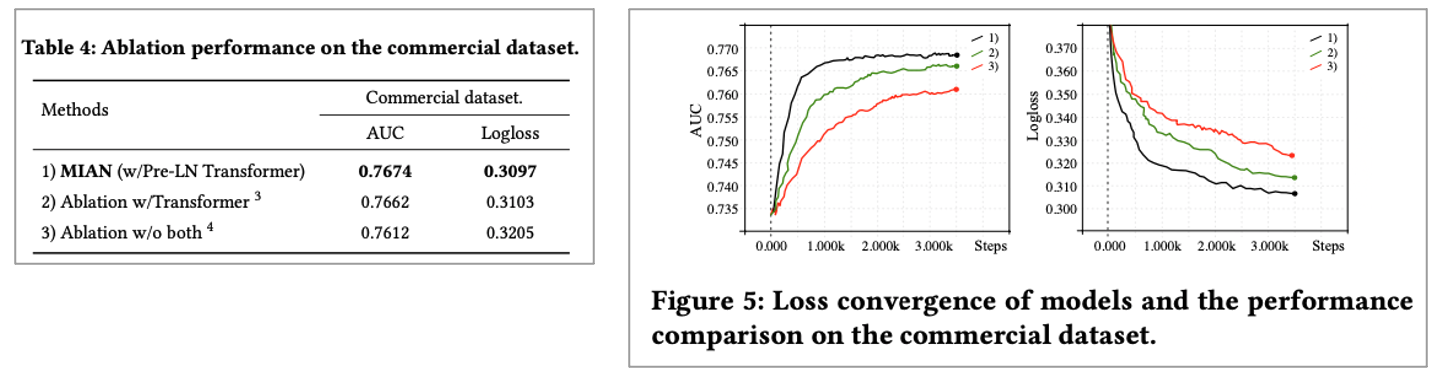
Transformer 방식을 제거하면 효과가 저하 되는 것을 확인할 수 있었으며 Original Transformer를 사용하면 User의 historical behavior간의 연관성을 추출하기 때문에 performance가 상승하는 것을 확인할 수 있었다고 합니다.
본 논문에서 사용한 Pre-LN Transformer는 안정적 + convergence speed를 상승하는 것을 확인할 수 있어서 Pre-LN 방식의 효과를 확인할 수 있었다고 합니다.
3. Visualization of Global attention
다음으로는 visualization study입니다. global attention을 기준으로 Fine grained interactive feature and interpretability of GIM을 직관적으로 보는 실험입니다. 이를 위해 random 하게 15개를 select해서 시각화해줍니다.
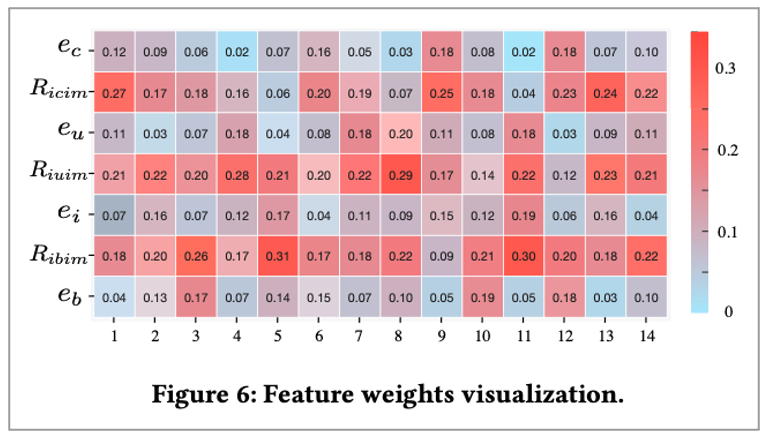
Ibim, uiim, icim은 original feature representation(e_c, e_u, e_i, e_b)에 비해 score가 더 크게 나오는 것을 확인할 수 있었으며 이는 High order feature interaction의 가능성을 시사한다고 합니다.
시각화 결과를 보면 fine grained feature learning의 중요성을 보여주며 MIAN은 Candidate item과 multiple fine grained information 사이의 deeply interactive association을 학습할 수 있다는 것을 보여준다고 합니다.
Conclusion
본 논문에서는 MIAN이라는 추천 시스템 모델을 소개합니다. MIAN은 CTR prediction의 performance 상승의 목적으로 다음과 같은 information 들을 사용합니다.
• Fine grained interaction among items, user sequential behavior, user specific and context information
또한, Multi Interactive layer를 디자인하고 이를 소개하였습니다. Pre-LN Transformer을 활용해 Effectively learn the fine grained interaction하였으며 Multiple local interactive modules을 활용하였습니다.
마지막으로 Global Interaction Module을 디자인하였습니다. 이것에 대한 특징은
• Capture the high order interaction between original feature and learned interactive representation
• And balance the different modules in a flexible way
으로 정리될 수 있습니다.
마무리
본 포스팅은 추천 시스템 논문 Multi-Interactive Attention Network for Fine-Grained Feature Learning in CTR Prediction (MIAN) 이라는 논문을 리뷰하는 포스팅입니다. 공부하시는데 조금이라도 도움이 되시길 바랍니다.
긴 글 읽어주셔서 감사합니다.